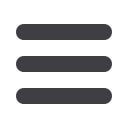
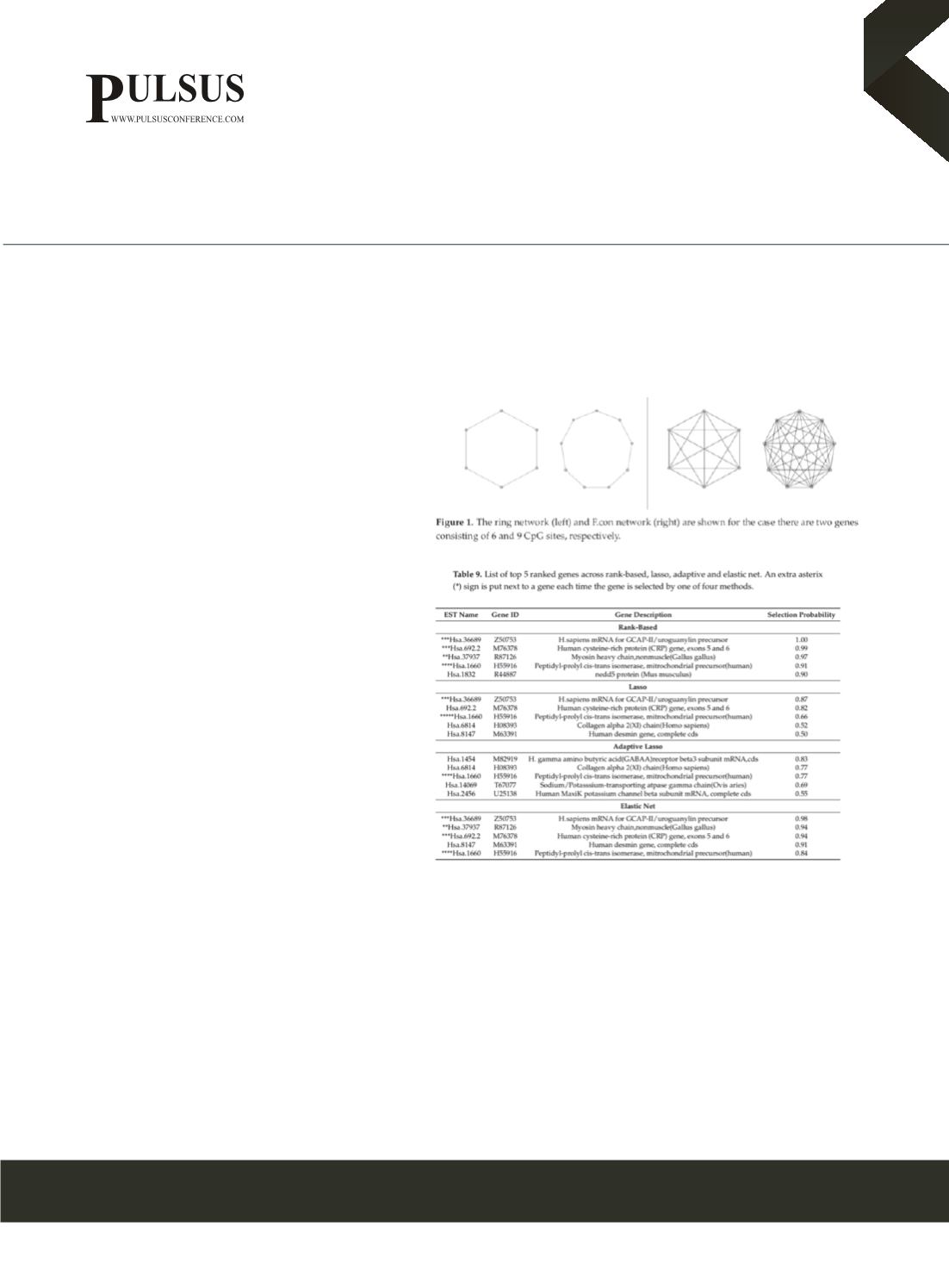
Page 36
Volume 3
August 5-6, 2019 | Singapore
CANCER RESEARCH AND PHARMACOLOGY
STRUCTURAL BIOCHEMISTRY, STEM CELLS AND MOLECULAR BIOLOGY
24
th
International Conference on
International Congress on
&
Cancer Research 2019 & Structural Biochemistry 2019
August 5-6, 2019
Journal of Cancer and Metastasis Research
On the performance of variable selection and classification via ranked based classifier
Showaib Rahaman Sarker
The University of Texas, USA
I
n high-dimensional gene expression data analysis,
the accuracy and reliability of cancer classification
and selection of important genes play a very crucial
role. To identify these important genes and predict
future outcomes (tumor vs. non-tumor), various
methods have been proposed in the literature. But
only few of them take into account correlation patterns
and grouping effects among the genes. In this article,
we propose a rank-based modification of the popular
penalized logistic regression procedure based on a
combination of l1 and l2 penalties capable of handling
possible correlation among genes in different groups.
While the l1 penalty maintains sparsity, the l2 penalty
induces smoothness based on the information from
the Laplacian matrix, which represents the correlation
pattern among genes. We combined logistic regression
with the BH-FDR (Benjamini and Hochberg false
discovery rate) screening procedure and a newly
developed rank-based selection method to come up
with an optimal model retaining the important genes.
Through simulation studies and real-world application
to high-dimensional colon cancer gene expression data,
we demonstrated that the proposed rank-based method
outperforms such currently popular methods as lasso,
adaptive lasso and elastic net when applied both to
gene selection and classification.
Biography
Showaib Rahman Sarker is pursuing his master’s degree in Statistics at The University of Texas at El Paso. He has expertise in
Statistical Machine learning application in High-Dimensional Gene Expression Data. Currently, he is doing his research in High through-
put cancer gene expression data. His main goal is to find out the important genes which are responsible for cancer and classify (tumor
vs non-tumor) accurately. He is passionate to apply statistical approach and machine learning approach in cancer research.
msarker@miners.utep.edu